Sampling is an important procedure in research and statistics, as it allows researchers to select part of the population to study. The two main approaches to sampling are random sampling and non-random sampling. The two approaches sharply contrast each other in terms of procedure, purpose, and outcome. Random sampling relies on probabilities whereby everyone in a population has an equal chance of being selected. In contrast, non-random sampling is subjective or based on convenience. These sampling techniques affect the reliability, validity, and applicability of the findings of the research. In this article, we go deeply to understand differences, their definitions, types, and when to use each.
Difference Between Random Sampling and Non-Random Sampling
Random sampling and non-random sampling are fundamentally different in their approach to selecting participants. Random sampling is rooted in probability, ensuring fairness and minimizing bias. It follows a structured approach that helps generalize results to the broader population. Conversely, non-random sampling focuses on practical and purposive methods, often involving the researcher’s discretion to target specific groups or individuals.
Aspect | Random Sampling | Non-Random Sampling |
---|---|---|
Selection Process | Based on probability methods. | Based on judgment, convenience, or quotas. |
Bias Risk | Low, due to equal selection chances. | High, due to selective inclusion. |
Generalizability | High; representative of the population. | Low; often specific to the study sample. |
Cost | Expensive due to resources required. | Cost-effective and resource-friendly. |
Time Requirement | Longer due to complex selection. | Quicker and simpler to implement. |
Defining Random Sampling
Random sampling ensures fairness and impartiality in picking people from a target population. It uses statistical tools and techniques that have a higher probability of member selection within the population to ensure that all members have the same chance of being picked. It, therefore reduces bias, hence ideal for scientific research and accurate data analysis.
For example, if a researcher wished to investigate the reading habits of university students, they might use random sampling by selecting individuals from the enrollment list in the university. This way, each student stands an equal chance of being selected, and the sample taken will therefore be representative.
Random sampling is essential for studies in which results must be able to reflect the larger population. For instance, in medical research, using random sampling helps establish whether a drug is effective generally across different population groups. It also reduces errors since the arbitrariness of the selection cancels out personal or systemic biases.
Despite these advantages, random sampling is very resource-intensive. Researchers need population data and tools to implement the selection process. Such can make the method time-consuming and expensive when dealing with huge populations.
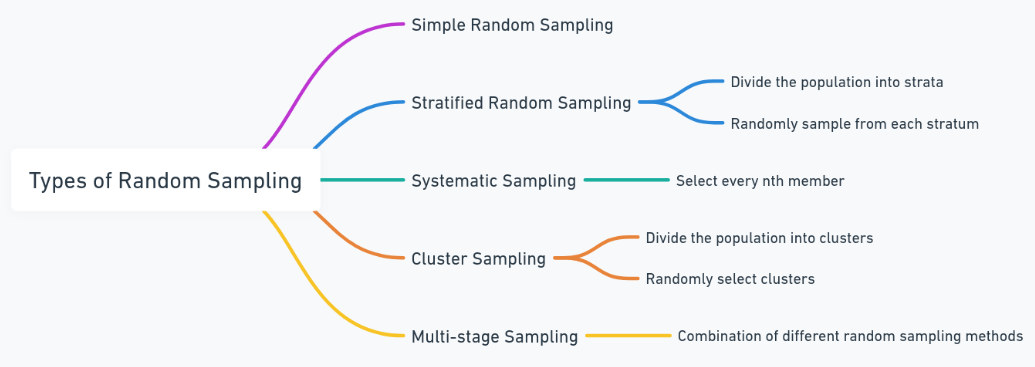
Types of Random Sampling
There are various types of random sampling, each suited to a particular type of research need. The type to choose depends on the size, diversity, and research objectives of a population.
- Simple Random Sampling: This is the most direct form. Researchers actually utilize random selection techniques, for instance, drawing names or generating numbers in creating a sample. For instance, drawing 100 employees from a company’s database ensures fairness since every employee has an equal chance of being selected.
- Stratified Sampling Stratified: It divides the population into distinct groups, or strata, according to shared characteristics such as age, income, or education. Then, a random sample of people is drawn within each stratum. This ensures that specific sub-groups are well-represented, making it ideal for use in studies among diverse populations.
- Systematic Sampling: In systematic sampling, every nth individual from the list or dataset is selected. It happens like this: a researcher can choose every 10th person in a line. Although it is systematic, it is still random and easier to implement for large populations.
- Cluster Sampling: In cluster sampling, the population is divided into clusters such as geographical areas or departments. Researchers randomly select some clusters and study all, or some participants in them. This method is also appropriate for large-scale studies where it would be impossible to access the whole population.
Defining Non-Random Sampling
Non-random sampling is often used in exploratory research where the focus would be on preliminary data rather than generalizing findings to an entire population. It allows researchers to target particular groups relevant to the study. For example, a researcher who aims to study the effects of a new teaching method can especially pick those teachers who have adopted it.
Although non-random sampling is open to a degree of bias compared to the random sampling procedure, which is not entirely objective. It is indeed efficient and practical, especially in cases where random sampling is infeasible either in terms of time, cost, or logistics.
For instance, while conducting a study on consumer behavior, nonrandom sampling may be used when interviewing shoppers at a particular mall. Though it is convenient, this method would omit people who shop elsewhere, thus limiting the generalizability of findings.
Types of Non-Random Sampling
Non-random sampling includes various methods designed to meet specific research goals. Each type has its advantages and limitations.
- Convenience Sampling: Convenience sampling occurs when sampling from participants through convenience and availability. Researchers may widely use this method for pilot studies or initial explorations. For instance, surveying friends or colleagues is considered convenience sampling.
- Quota Sampling: Quota sampling ensures that certain groups are represented in specific proportions. Researchers set quotas for different categories, such as gender or age, and select participants until the quotas are filled. Though structured, this method is reliant on non-random criteria.
- Purposive Sampling: Purposive sampling involves choosing participants based on their appropriateness to the research issue. For example, for a study on technology adoption, it would be appropriate to select IT professionals in particular.
- Snowball Sampling: The process begins with a few participants who then go on to recruit others from their networks. Therefore, it is advantageous where hidden or difficult-to-reach populations are under research. These include those who suffer from rare medical conditions.
Conclusion
Understanding the difference between random sampling and non-random sampling is very important because it helps one choose the correct method appropriate for research. Random sampling is desirable for studies that need unbiased generalizable results, while non-random sampling is applicable for exploratory or resource-constrained research. Each has strengths and limitations, and the choice depends on the objectives of the research, population characteristics, and available resources.
Random vs Non-Random Sampling FAQs
What is the primary distinction between random and non-random sampling?
Random sampling ensures every individual has an equal chance of selection, reducing bias. Non-random sampling relies on subjective or convenience-based criteria, often introducing bias.
Why is random sampling considered more reliable?
It minimizes bias and ensures that the sample represents the population, making results more generalizable.
Can random and non-random methods be combined?
Yes, mixed sampling methods can be used to balance practicality and generalizability, such as using non-random methods for initial selection and refining with random techniques.
When should non-random sampling be used?
Non-random sampling is ideal for exploratory studies, limited resources, or targeting specific, hard-to-reach groups.
Is systematic sampling considered random or non-random?
Systematic sampling is a type of random sampling as it uses regular intervals to maintain randomness in participant selection.